Deep Learning for Patch Clamp Electrophysiology
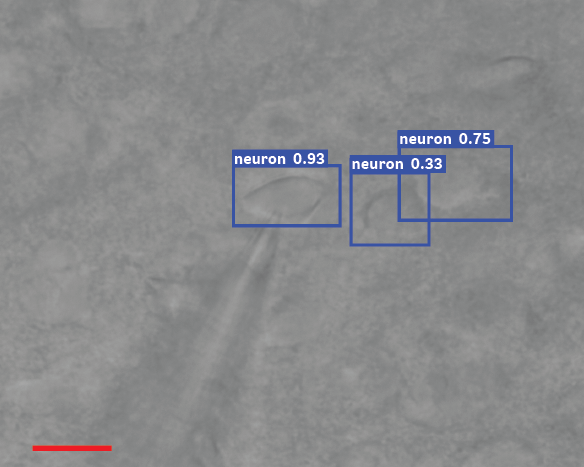
Scale bar represents 10 µm. Image courtesy of Mighten C. Yip
With the recent advances in deep learning enabling rapid and accurate identification of complex structures, it’s application to in vitro patch-clamp electrophysiology was inevitable. We are exploring the application of these techniques to enhance the capabilities of our fully-automatic patch clamp robots. For example, we have demonstrated fully automatic, real-time detection of healthy neurons within traditional DIC images and vision-based techniques to correct for hardware error stack-up during pipette localization.
Related Publications
Gonzalez, M. M., Lewallen, C. F., Yip, M. C., & Forest, C. R. (2021). Machine Learning-Based Pipette Positional Correction for Automatic Patch Clamp In Vitro. ENeuro, 8 (August), 1–8. doi: https://doi.org/10.1523/ENEURO.0051-21.2021
[PDF]M.C. Yip, M.M. Gonzalez, C.R. Valenta, M.J.M. Rowan, C.R. Forest. Deep learning-based real-time detection of neurons in brain slices for in vitro physiology. Sci Rep 11, 6065 (2021). https://doi.org/10.1038/s41598-021-85695-4
[PDF]M.M. Gonzalez, M.C. Yip, C.F. Lewallen, M.J. Rowan, C.R. Forest, Machine learning-based pipette correction for automated patch clamp in vitro, SfN Global Connectome, Virtual Conference, Jan 11-13, 2021.